Generative AI, once labelled as the next revolution in enterprise technology, has hit a rough patch. According to Gartner, many organizations find themselves navigating what can be called the “Trough of Disillusionment.” The initial excitement surrounding large language models (LLMs), and their generative capabilities has given way to a more sober reality—results are not matching expectations. Enterprises are grappling with deployment challenges, underwhelming outcomes, and the stark realization that broad, one-size-fits-all AI models are not living up to the promise.
But disillusionment is not defeat. In fact, the path forward is becoming clearer. As organizations refine their use of AI, specialized models, and complementary technologies such as graph-enhanced retrieval-augmented generation (RAG) are emerging as practical solutions to bridge the performance gap.
Why are we in the trough?
The hype surrounding generative AI was driven by its potential to revolutionize industries, automate content creation, and improve decision-making. But this potential came with a set of assumptions—that LLMs trained on vast datasets could effortlessly generalize to any context, and that deploying AI would immediately yield productivity gains. However, as Gartner highlights, many enterprises have hit significant roadblocks.
Performance inconsistency is the primary challenge. While LLMs excel at generating human-like text, they often lack the domain-specific accuracy needed for nuanced business tasks. Enterprises need answers to specific questions, but generic models often deliver incomplete or irrelevant results. Moreover, the scale of LLMs introduces operational complexities, from computational costs to integration hurdles.
These shortcomings have led many to question whether GenAI is ready for prime time. But the real issue is not the technology itself, it is the misalignment between expectations and practical applications. The solution lies in a more specialized, targeted approach.
Specialized LLMs
Specialized large language models (LLMs) are a targeted solution designed to address the performance gap. Unlike general-purpose models, specialized LLMs are fine-tuned for specific industries, use cases, or even individual companies. By focusing on a narrower dataset and a defined task, these models offer superior performance, delivering more accurate and contextually relevant results.
For example, a healthcare focused LLM trained specifically in medical literature and terminology can provide more precise diagnostic insights than a generic model trained on vast, unrelated data. Similarly, an LLM tailored for financial services will understand industry-specific regulations, market trends, and client data, allowing for better risk assessment and compliance automation.
The key to making the most of GenAI lies in customization. Instead of relying on a one-size-fits-all model, enterprises should invest in developing and training specialized LLMs that can truly address their unique needs.
Graph-Based Retrieval-Augmented Generation (RAG)
Another breakthrough in closing the performance gap is graph-enhanced retrieval-augmented generation (RAG). While traditional RAG systems leverage vectors to retrieve relevant data from knowledge bases, graph-based RAG takes it a step further by mapping and utilizing the relationships between data points.
In a graph-enhanced RAG system, entities (e.g., products, customers, or business processes) are represented as nodes, and their relationships (e.g., dependencies, transactions, interactions) are edges. This allows the model to retrieve similar data points and contextually relevant ones based on how they are interconnected.
This approach dramatically improves contextual accuracy. Rather than a flat retrieval from a database, graph-based RAG provides a rich, multi-dimensional view of information. This is particularly useful in complex industries such as supply chain management, where understanding the relationship between suppliers, products, and logistics is critical to decision-making.
Integrating graph technology with GenAI bridges the gap between generic outputs and actionable insights, enabling businesses to navigate complex environments with greater precision.
Closing the Performance Gap
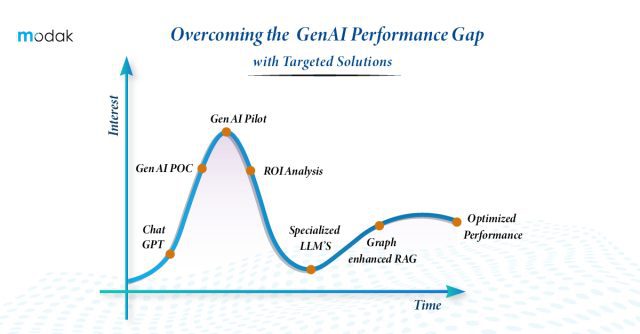
As organizations move through the Trough of Disillusionment, it is important to shift the narrative. GenAI is not underperforming because the technology is flawed; it is struggling because it is being applied too broadly. The way forward is to adopt a more focused approach, one that integrates specialized LLMs, and graph enhanced RAG to solve the real, nuanced challenges enterprises face.
Here’s how businesses can start to close the GenAI performance gap:
1. Identify specific use cases: Do not deploy generative AI across the entire enterprise. Instead, focus on high-value, clearly defined use cases where AI can make a measurable impact. Whether it is automating customer support in a specific industry or optimizing procurement processes, narrow down the scope to ensure better results.
2. Invest in specialized models: Off-the-shelf LLMs are not the answer for every business. Enterprises should invest in customizing or fine-tuning models that understand their industry, business processes, and specific pain points. By tailoring models to their needs, companies will see more relevant and reliable outcomes.
3. Leverage graph technology: For industries that rely heavily on understanding relationships and dependencies, integrating graph-based RAG can significantly enhance the contextual accuracy of AI outputs. This approach goes beyond simply retrieving data; it retrieves data that is meaningfully connected to the task at hand.
4. Partner with the right expertise: Building specialized AI solutions requires deep technical expertise. Enterprises should consider partnering with companies that have experience in both AI development and the specific technologies like graph databases that can optimize their performance.
The trough of disillusionment is not the end of the GenAI story, it is a turning point. For enterprises, this phase represents an opportunity to refine their AI strategies and adopt solutions that are better aligned with their needs. Specialized LLMs and graph-enhanced RAG systems are key components of this new approach, offering more precision, context, and relevance than ever before.