In the world of research and development, one of the biggest challenges scientists face is how to make sense of vast amounts of data. For a top five life sciences company in the U.S., this problem became a barrier to accelerating drug discovery and optimizing research processes. The question wasn’t about gathering data—they had plenty. The real challenge was finding meaningful insights by connecting data from multiple, often siloed, sources. This is where advanced graph technology came into play, offering a powerful alternative to traditional databases.
Our client, a leader in life sciences, was struggling with fragmented datasets—ranging from clinical trials and genome studies to patent filings and research papers. Traditional relational databases couldn’t handle the complexity or reveal the hidden relationships between the data. They needed a more flexible solution that could connect both structured and unstructured data sources and allow their R&D teams to explore relationships in real-time.
The solution came in the form of Neo4j where, instead of storing data in rigid tables, Neo4j captured data as nodes and relationships, offering a more intuitive way to model and query complex datasets. What does this mean in practice? For scientists working with drug compounds and disease pathways, graph technology enables them to instantly visualize how different entities are connected. This dramatically reduces the time spent querying the data, allowing them to focus on analyzing potential drug interactions, adverse events, and genomic correlations.
But adopting a new data platform isn’t just about switching technology. The success of graph analytics depends on how quickly and efficiently data can be prepared and loaded into the system. That’s where Modak’s Nabu comes into play.
Modak Nabu™ automates the process of ingesting, preparing, and orchestrating data. It transforms complex datasets—both structured and unstructured—into a format that can be easily consumed by Neo4j. By streamlining this data preparation, Nabu cuts down on manual effort and ensures that the client’s R&D teams have clean, ready-to-use data at their fingertips.
Our pipelines feed data directly into Neo4j, enabling the client to unlock the maximum potential of graph analytics. With Modak Nabu™ working behind the scenes, researchers no longer have to spend months waiting for their data to be ready for analysis. Instead, they can quickly discover hidden relationships and patterns that were previously obscured by data silos.
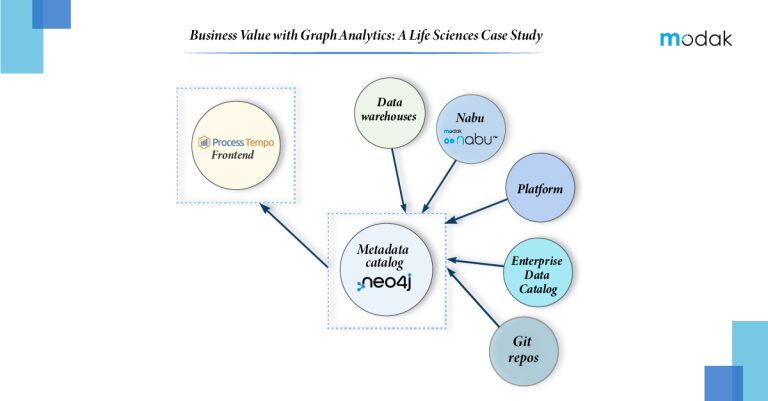
Business Impact?
The switch to graph analytics wasn’t just a technical change—it led to measurable business outcomes for the client. By combining the power of Neo4j with Modak’s Nabu, the client saw a significant reduction in time to value. Previously, it could take up to 12 months to prepare data and generate meaningful insights. With the new solution in place, this process was accelerated by 4x, cutting the timeline to just three months.
Additionally, the new system enabled the R&D team to reduce costs by 40%—a direct result of better data orchestration, faster insight generation, and reduced manual intervention.
But more importantly, the adoption of graph technology enabled their researchers to ask bigger, more strategic questions. With Neo4j, they could explore new hypotheses, investigate drug interactions faster, and potentially reduce the time it takes to bring a drug to market.
In addition to Modak and Neo4j, another partner played a key role in this transformation: Process Tempo. They ensured that the insights generated by the client’s R&D teams were effectively translated into actionable business strategies. By providing real-time visibility into data workflows, Process Tempo added an extra layer of efficiency to the overall process.
The switch to graph analytics wasn’t just a technical change—it led to measurable business outcomes for the client. By combining the power of Neo4j with Modak’s Nabu, the client saw a significant reduction in time to value. Previously, it could take up to 12 months to prepare data and generate meaningful insights. With the new solution in place, this process was accelerated by 4x, cutting the timeline to just three months.
Additionally, the new system enabled the R&D team to reduce costs by 40%—a direct result of better data orchestration, faster insight generation, and reduced manual intervention.
But more importantly, the adoption of graph technology enabled their researchers to ask bigger, more strategic questions. With Neo4j, they could explore new hypotheses, investigate drug interactions faster, and potentially reduce the time it takes to bring a drug to market.
In addition to Modak and Neo4j, another partner played a key role in this transformation: Process Tempo. They ensured that the insights generated by the client’s R&D teams were effectively translated into actionable business strategies. By providing real-time visibility into data workflows, Process Tempo added an extra layer of efficiency to the overall process.

Why is Graph Analytics a Game-Changer?
Traditional relational databases, while still useful for certain applications, fall short when it comes to understanding relationships within data. Their rigid structure is not built to handle the complexity of modern data landscapes. This is especially true for organizations in life sciences, where data spans everything from clinical research to real-world evidence.
Graph databases like Neo4j offer a different approach. By visualizing data as nodes and connections, they allow users to explore relationships dynamically. This flexibility is crucial when analyzing complex datasets such as gene-disease associations, drug interactions, or patient health records.
For our client, the move to graph technology was a natural evolution of their data strategy. It enabled them to move beyond basic data-driven insights and towards a more intelligence-driven approach, where data relationships are explored at the speed of thought.
Looking ahead, the possibilities for graph analytics are endless. As more organizations adopt this technology, we expect to see even greater advancements in areas like personalized medicine, clinical trial optimization, and drug discovery.