In the bustling headquarters of a thriving multinational corporation, resided Mr. X, a highly regarded senior manager renowned for his exceptional leadership skills and strategic acumen. With years of experience under his belt, he was trusted implicitly with critical decision-making and the company's most valuable asset- data. While working on a crucial report to understand the clinical trials data for a specific drug discovery, unknown to Mr. X, lurking within the depths of the data was a discrepancy that was missed during the initial analysis. A minor glitch in data extraction had caused a miscalculation, leading to an inflated projection of data.
As the blunder slowly emerged, the blame fell on Mr. X. The senior manager, once regarded as a beacon of expertise, found himself at the center of a storm, grappling with the harsh consequences of a data quality blunder. In the aftermath, the organization was forced to remove Mr. X from his position, reassess its data governance policies, implement stringent data quality measures, and invest in advanced data analytics tools to prevent such incidents from occurring in the future.
Despite the unfortunate outcome of Mr. X's experience, his story is not an isolated incident. In fact, data quality issues are pervasive in today's data-driven landscape, affecting organizations across industries and of all sizes. The implications of data quality mishaps can be far-reaching and devastating, leading to erroneous decisions, lost opportunities, damaged reputation, and significant financial losses. As businesses increasingly rely on data to gain a competitive edge and respond to dynamic market conditions, the need for accurate, reliable, and high-quality data becomes paramount.
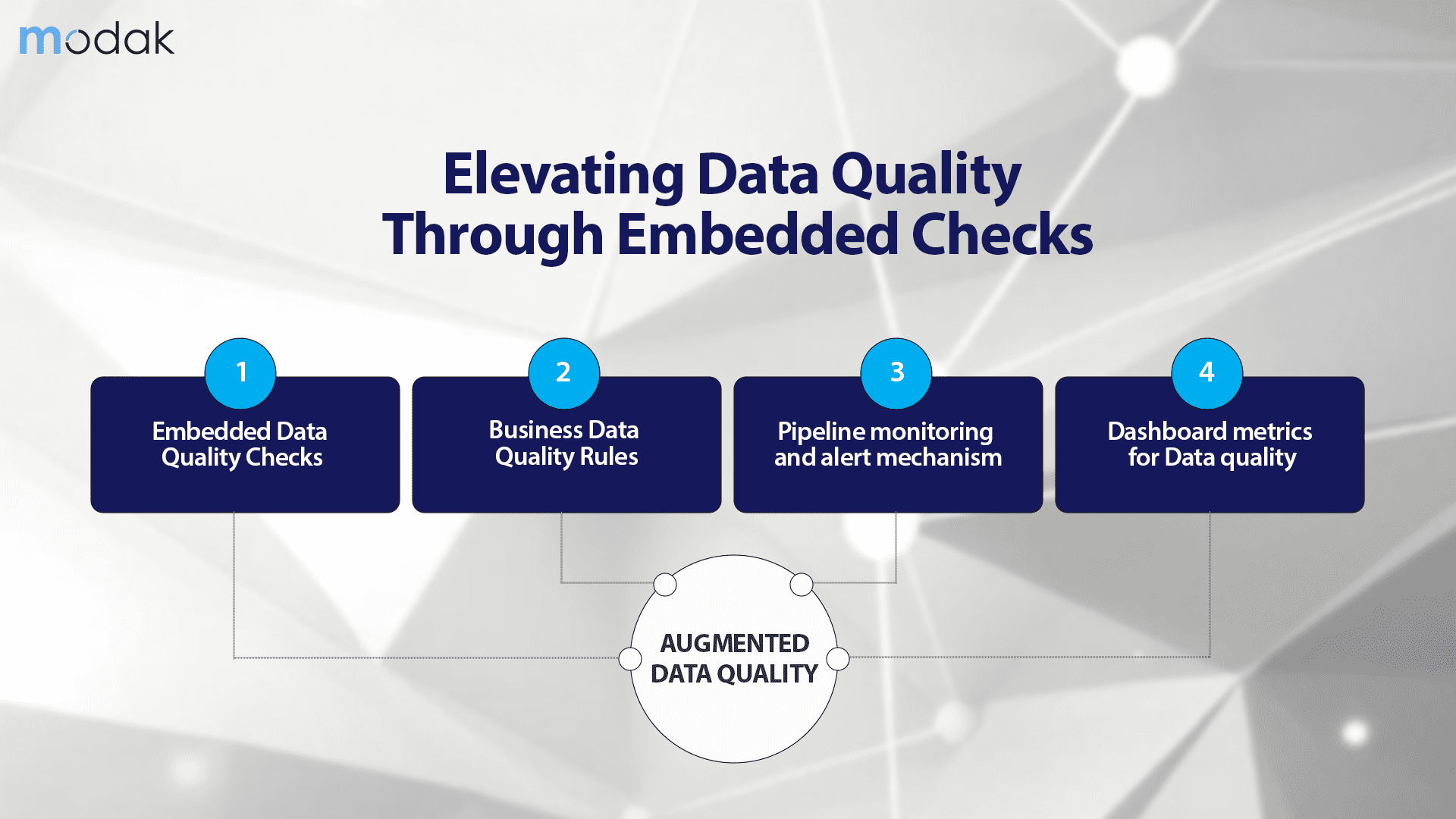
Data Quality can’t be an Afterthought
Embedded Data Quality Rules into Data Pipelines
Business-specific Rules and Thresholds
Implementing Alert Mechanisms
PII and Governance Process
Schema Change/Drift and AI-Based Rules
Conclusion
About Modak
Modak is a solutions company dedicated to empowering enterprises in effectively managing and harnessing their data landscape. They offer a technology, cloud, and vendor-agnostic approach to customer datafication initiatives. Leveraging machine learning (ML) techniques, Modak revolutionizes the way both structured and unstructured data are processed, utilized, and shared.
Modak has led multiple customers in reducing their time to value by 5x through Modak’s unique combination of data accelerators, deep data engineering expertise, and delivery methodology to enable multi-year digital transformation. To learn more visit or follow us on LinkedIn and Twitter.