“Dirty Data” is the biggest challenge to overcome in Machine Learning, according to a 2017 survey by Kaggle with over 16,000 data scientists.
This statistic underscores the pervasive challenges data silos create for businesses. Today, industries across the globe find themselves impeded by their siloed data, hindering their ability to tap into the full potential of advanced technologies such as Artificial Intelligence (AI) and Machine Learning (ML). This is where FAIR-driven data comes into play.
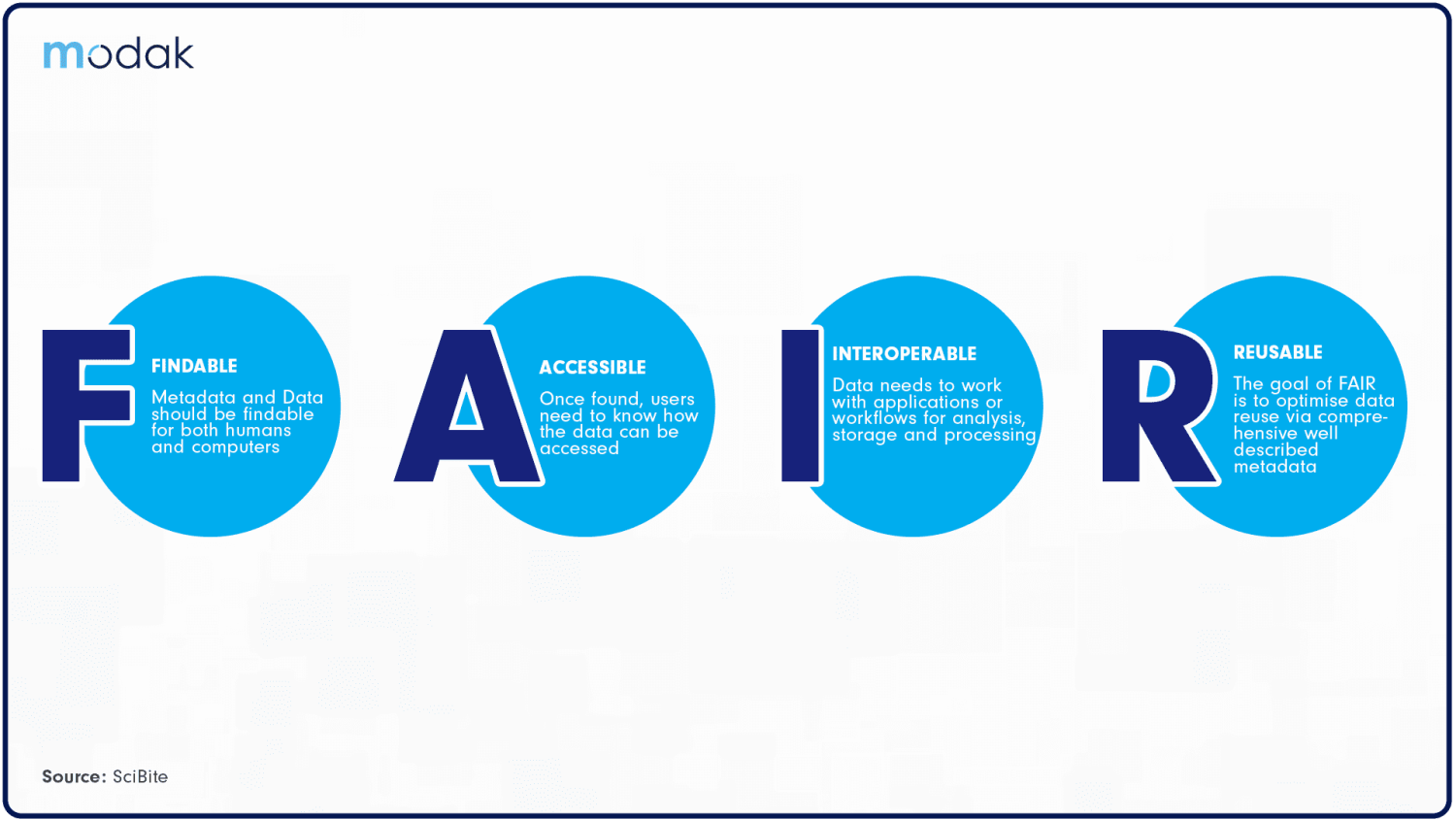
The FAIR Framework: A Universal Solution
Understanding FAIR-Driven Platforms
In practical terms, this means FAIR-driven data platforms seamlessly blend data from various sources, such as sales, marketing, and production, into a unified ecosystem. This integration creates a comprehensive organizational view, transcending individual departmental boundaries. As a result, businesses can make data-driven decisions, breaking free from the limitations imposed by data silos, and harnessing the full potential of their information assets..
Enhancing AI/ML with FAIR Data
Furthermore, FAIR data leverages ontologies, and structured knowledge models expediting the learning process for AI models. These ontologies provide AI models with a structured foundation of domain knowledge, significantly expediting the learning process. Consider the example of an ontology, encoding the relationship between "Concept Z" and "Attribute A." AI models can swiftly grasp this connection, significantly enhancing their accuracy and efficiency. FAIR data doesn't just enhance AI/ML training; it also provides high-quality data inputs necessary for accurate results in applications like sentiment analysis and anomaly detection.
Empowering Search with FAIR Data
FAIR data goes a step further by incorporating deep learning techniques into the mix. Deep learning equips modern search engines with the ability to discern the intent behind a query, similar to everyday search engines. This transformative capability empowers users to employ natural language queries, opening doors to a treasure trove of information. Complex questions, such as predicting market trends or customer behavior, become accessible and solvable through the power of FAIR data-driven platforms.
The Benefits of FAIR Data-Driven Platforms
FAIR data-driven platforms bring several advantages, transforming data into a strategic asset. These benefits encompass:
- Improved Data Quality: Enhance data quality by ensuring proper documentation and tagging. This meticulous approach simplifies data discovery and utilization while minimizing errors.
- Increased Data Accessibility: Establish a centralized repository for data, equipped with robust search and access tools. This accessibility ensures businesses can swiftly locate the data they require, regardless of its location.
- Enhanced Data Interoperability: Promote data interoperability by enforcing consistent formats and standard metadata tags. This seamless integration facilitates data sharing across diverse systems and applications.
- Increased Data Reusability: Augment data reusability through comprehensive documentation and tagging. This enables data to be repurposed effectively for various applications, including machine learning and analytics.
Summary
About Modak
Modak is a solutions company dedicated to empowering enterprises in effectively managing and harnessing their data landscape. They offer a technology, cloud, and vendor-agnostic approach to customer datafication initiatives. Leveraging machine learning (ML) techniques, Modak revolutionizes the way both structured and unstructured data are processed, utilized, and shared.
Modak has led multiple customers in reducing their time to value by 5x through Modak’s unique combination of data accelerators, deep data engineering expertise, and delivery methodology to enable multi-year digital transformation. To learn more visit or follow us on LinkedIn and Twitter.