Generative AI (Gen AI) has garnered significant attention in recent years across various industries. Enterprises are actively exploring ways to leverage the power of Gen AI for improving their operations and user experience at the earliest. In fact, a recent study by McKinsey revealed that Generative AI (GenAI) will add $2.6 Trillion to $4.4 Trillion to the value of economy. However, it is also estimated that 90% of all data in the future will be unstructured.
Gen AI presents a plethora of potential for industries that are yet to be explored. Nonetheless, harnessing GenAI’s capabilities poses a significant challenge of avoiding pitfalls that often plague custom GenAI models, the primary one being data preparation. Traditional data integration platforms fall short in preparing unstructured data for LLMs or GenAI training. It highlights the need for meticulous preparation of data with the right tools and processes for this unique task.
Fortunately, with the influx of GenAI, data management tools have undergone significant upgrades for catering to the foundational needs of custom AI platforms. These tools with appropriate strategies can contribute to successful development and implementation of Generative AI for enterprises.
Challenges of Custom Gen AI Implementation
Although the potential benefits of custom Generative AI are enticing, its integration into enterprise systems poses significant challenges.
- Decision-making process: Large organizations may have bureaucratic structures that impede swift adoption of new technologies like generative AI.
- Data Quality: Fragmented or incomplete data sets can limit the effectiveness of generative AI models, requiring extensive cleaning and preprocessing efforts.
- Incentives: Conflicting priorities among stakeholders may result in resistance or lack of support for generative AI initiatives within the enterprise.
- Complex ERP Applications: Complex enterprise resource planning (ERP) systems may present challenges for integrating generative AI due to concerns about disruption or compatibility issues.
Essential Capabilities for Generative AI Implementation
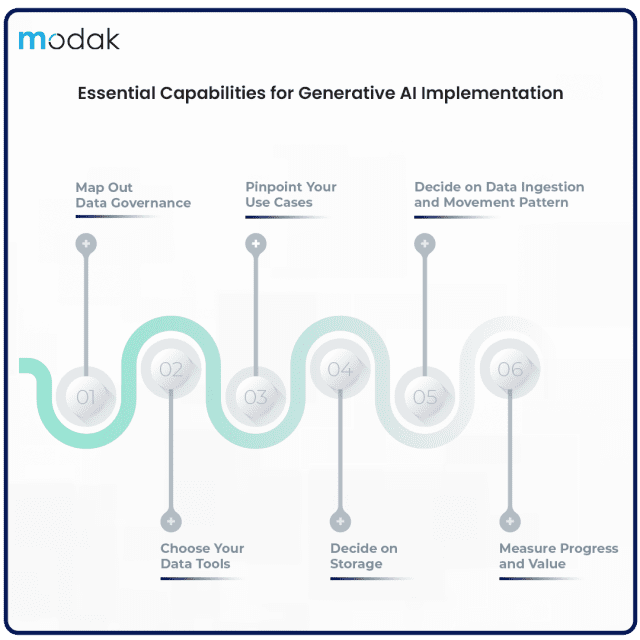
Map Out Data Governance
Before diving into GenAI initiatives, enterprises should establish a robust data governance policy tailored to their data and business needs. This includes acquiring tools for tracking data lineage, ensuring transparency in data flows feeding into AI models, and prioritizing the handling of sensitive data and PII with automatic detection and hashing capabilities. Metadata tagging and tracking are also crucial for finding and tracking data movement and lineage.
Choose Your Data Tools
Data is the lifeblood of GenAI, and companies should select the right tools carefully. Look for tools that offer flexibility and can handle personally identifiable information (PII) for use cases involving private or sensitive data. Purchasing flexible tools designed for the job can often save time and money compared to building in-house from scratch.
Pinpoint Your Use Cases
Rather than taking a broad approach, enterprises should carefully select and plan for specific use cases, tailoring their AI initiatives to deliver targeted solutions. Such an approach can maximize the impact and return on investment of their AI implementation. It also helps in setting clear goals and expectations for the AI project, enabling better planning and resource allocation.
Decide on Storage
At an enterprise scale, enterprises should choose the right data storage solution. Considerations should include the ability to handle unstructured data efficiently, support real-time updates if necessary, and enable easy discoverability and collaboration. Metadata tagging and cataloging are essential for tracking data journeys and facilitating data accessibility for teams.
Decide on Data Ingestion and Movement Pattern
Enterprises should plan and acquire tools and platforms to move data efficiently between storage, producers, and AI models. Traditional ETL/ELT platforms may struggle with unstructured data, so choose a platform that can handle this complexity and connect with all data storage solutions while supporting the speed requirements of use cases.
Measure Progress and Value
Companies should monitor GenAI's impact to demonstrate its value to stakeholders and provide insights for future AI experiments. Continued measurement helps identify the most valuable components within a company's data processes for AI.
Key Takeaways
At Modak, we believe that the future of data-driven decision-making lies in the collaboration between data engineering and GenAI. We are passionate about helping organizations unlock the potential of this powerful combination. If you're ready to take your data initiatives to the next level, contact Modak today. Let's discuss how we can help you leverage the power of data engineering and GenAI to achieve your business goals.
About Modak
Modak is a solutions company dedicated to empowering enterprises in effectively managing and harnessing their data landscape. They offer a technology, cloud, and vendor-agnostic approach to customer datafication initiatives. Leveraging machine learning (ML) techniques, Modak revolutionizes the way both structured and unstructured data are processed, utilized, and shared.
Modak has led multiple customers in reducing their time to value by 5x through Modak’s unique combination of data accelerators, deep data engineering expertise, and delivery methodology to enable multi-year digital transformation. To learn more visit or follow us on LinkedIn and Twitter.