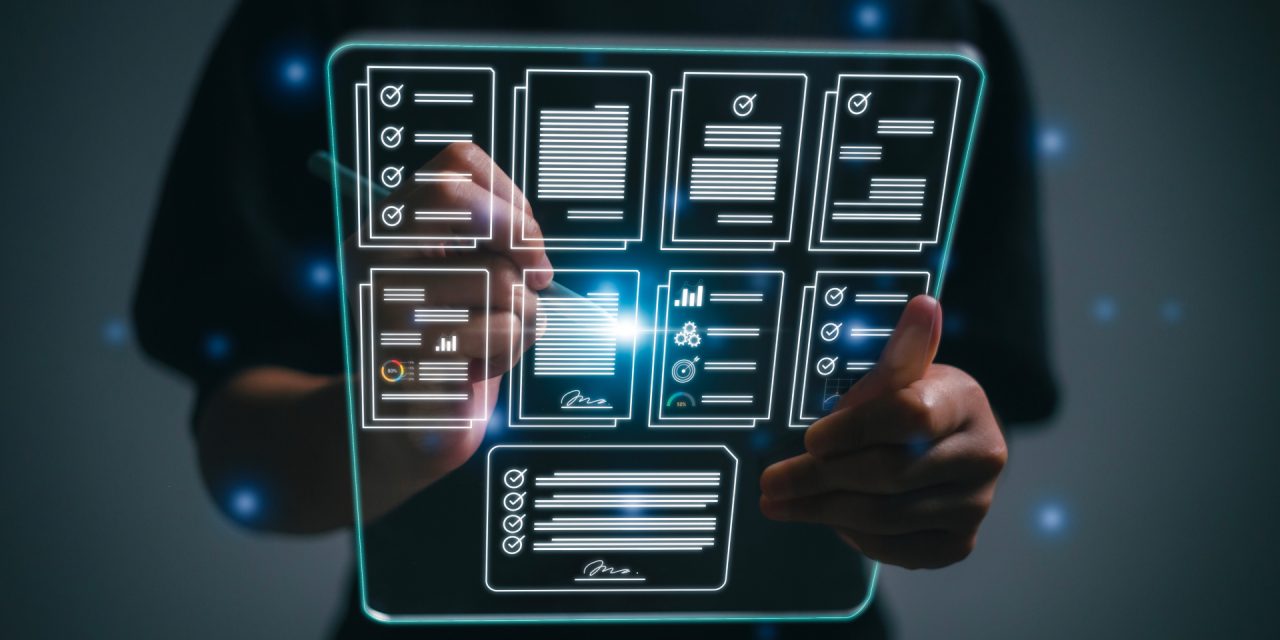
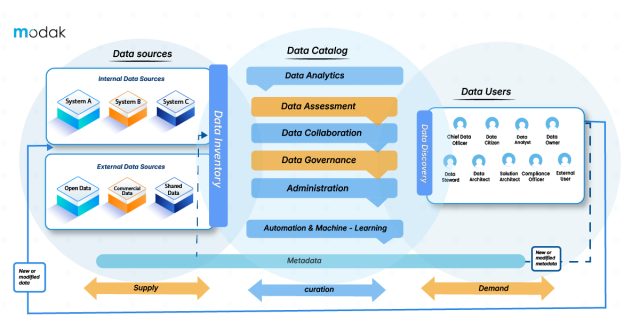
The digital transformation has led to a massive surge in both the quantity and diversity of available data. This represents an outstanding opportunity for different organizations for whom data has been an integral part of their service and product portfolio. However, since we rely on AI to make sense of big and complicated datasets, one important aspect of modern data management is getting renewed attention: Data Catalog. Firms with effective data catalog utilization witness awesome changes in the quality and speed of data analysis and in the interest and engagement of people who want to perform data analysis.
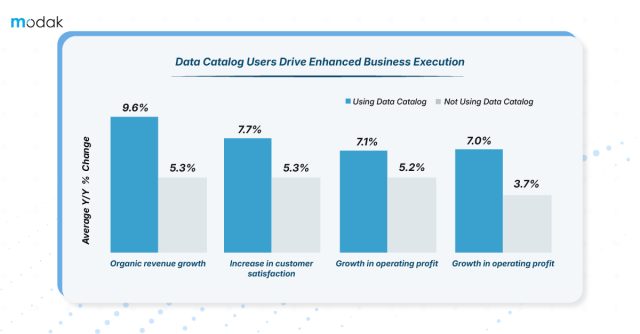
The Essentials of Data Cataloging and Metadata Management
According to the Aberdeen’s research, firms deal with data environments that are developing in excess of 30% every year, some much better than that. Data catalog help data teams to locate, comprehend, and implement data more effectively by organizing data from different sources on a centralized platform
The Essentials of Data Cataloging and Metadata Management
In this data-driven age, streamlined data management is not just an option- it’s a necessity. Efficient data cataloging and metadata management enable businesses increase operational efficacy, comply with strict regulations, and get actionable insights from their data.
Decoding Data Cataloging
Data cataloging is the systematic organization of data into a searchable repository, much like books in a library. This system allows businesses to efficiently locate, understand, and utilize their data assets.
“A data catalog creates and maintains an inventory of data assets through the discovery, description, and organization of distributed datasets. The data catalog provides context to enable data stewards, data/business analysts, data engineers, data scientists and other lines of business (LOB) data consumers to find and understand relevant datasets for the purpose of extracting business value. Modern machine-learning-augmented data catalogs automate various tedious tasks involved in data cataloging, including metadata discovery, ingestion, translation, enrichment and the creation of semantic relationships between metadata. These next-generation data catalogs can, therefore, propel enterprise metadata management projects by allowing business users to participate in understanding, enriching, and using metadata to inform and further their data and analytics initiatives.’’
– Gartner, Augmented Data Catalogs 2019. (Access for Gartner subscribers only)
The Role of Metadata
Now we are clear about data catalogs- data management, searching, data inventory, and data evaluation- but all rely on the core potential to offer a collection of metadata.
What is Metadata?
Ideally, metadata is the data that offers proper information about other data. We can say that metadata is “data about data”. It has markers or labels that describe data, making it seamless to understand, identify, organize, and use. Metadata can be implemented with different data formats, utilizing images, documents, databases, videos, and more.
In addition to the significance of data cataloging and metadata, data quality plays an important role in data management. Data quality efforts can be improved greatly by properly cataloging your data. When metadata gives context and structure, it becomes easier to recognize redundancies, inconsistencies, or gaps in data, permitting businesses to enhance their data quality initiatives. Data cataloging and quality advancements ensure that firms not only understand their data better but also trust and implement it more efficiently by working hand-in-hand.
Metadata management involves handling data that describes other data, providing essential context about the data’s content and purpose. It acts like a quick reference guide, enabling users to understand the specifics of data without delving into the details.
Understanding Metadata in the AI Era
Metadata acts as the cornerstone of data management strategy. It offers structure, context, and insightful meaning to raw data, helping systems and users to search, understand, and use information very efficiently. Previously, metadata was normally utilized to index and retrieve data in databases and file systems. However, with the advancement of machine learning and artificial intelligence, the role of metadata has emerged effectively.
One of the main challenges that enterprises face is maintaining the consistency and accuracy of metadata over time, specifically as data evolves. Traditionally, data stewards were responsible for managing and updating metadata manually, a procedure that was both prone to errors and labor-intensive. This results in inefficiencies, specifically in large-scale operations where data complexity is greater.
With the emergence of AI-driven cataloging strategies, these difficulties are being fixed more efficiently. ML algorithms can generate, extract, and enrich metadata automatically, reducing the manual work required to maintain data catalogs. This helps businesses to scale their data operations, ensure regular updates, and improve the quality of metadata management. AI helps automate processes like metadata classification, data tagging, and even the identification of relationships between datasets, minimizing the requirement of extensive manual intervention
The Role of Data Cataloging to Improve AI Capabilities
Data cataloging is the process of creating an organized inventory of data assets within an enterprise. It encompasses documenting metadata including data resources, relationships, formats, and usage regulations, in a central repository. For data assets, data catalogs act as a single source of truth, offering users an inclusive view of available data and its related metadata.
Previously, utilizing data cataloging has been an issue for firms because of the complications of handling huge data spread across several systems. Manually keeping metadata updated by data stewards was mostly prone to human error and time-consuming. Furthermore, fragmented data made it challenging to achieve true interoperability, resulting in inefficiencies and incomplete insights.
However, now AI is revolutionizing how data cataloging is used, mitigating the dependency on manual procedures. With the emergence of AI and automation, firms can now manage data at scale, generate metadata automatically, and decrease the requirement for frequent human intervention. This move not only ensures that data is updated and standardized continuously but also boosts the accuracy and speed of data discovery significantly.
One of the vital advantages of data cataloging is better data discoverability. In big enterprises, data is scattered across many databases, systems, and departments. This fragmentation makes it difficult for users to identify the data they require, resulting in inefficiencies and missed opportunities. A well-curated data catalog addresses this problem by offering a searchable data index of data assets, complete with comprehensive metadata that describes every dataset’s origin, content, and relevance. Not only does this make it effortless for users to identify the data they require but also helps AI systems to access and process data more effectively.
Furthermore, data cataloging improves data compliance and governance. In today’s environment, enterprises must ensure that their data practices comply with numerous rules and regulations. Data catalogs enable enterprises to maintain prominence and control over their data assets, helping them to track data lineage, enforce data government policies, and monitor data usage. Specifically, this level of oversight is significant in different AI applications, where the capability for bias and ethical concerns is paramount. Enterprises can ensure that their AI systems operate ethically and transparently by cataloging metadata and documenting data resources.
The Effect of AI on Metadata Management
As AI is evolving continuously, it’s changing the way metadata is handled. Usually, metadata management is a time-consuming process, need data stewards to document accurately and update metadata for every dataset. However, with AI, organizations are now able to streamline this process, automating much of the metadata generation and management.
One of the most important developments in this area is the implementation of AI to generate and enrich metadata automatically. This has led to a shift in how organizations scale their data management capabilities. The algorithms of machine learning can analyze different datasets to extract relevant metadata like relationships, data types, and strategies. This not only reduces the burden on data stewards but also ensures that metadata is updated continuously as new data is ingested. Furthermore, artificial intelligence can be utilized to find and resolve metadata inconsistencies like wrong or missing information, further improving the reliability and accuracy of data catalogs.
Enterprises can scale their data operations while ensuring that metadata remains actionable and correct across multiple datasets by adopting AI-driven automation. In metadata management, the role of AI is not only regarding efficacy but also regarding exploring the capability for real-time, scalable data cataloging that supports enterprise-wide decision-making at a larger scale than was possible previously.
Also, AI-powered metadata management helps with more advanced data discovery and analytics. For instance, natural language processing (NLP) techniques can be implemented to metadata to enable more context-aware and intuitive search abilities. Users can search for data using natural language queries, and AI algorithms can understand the objective behind the query and find the most relevant data assets. This makes data discovery easier for non-technical users and improves data catalog usability.
Another evolving trend is AI usage to improve data lineage tracking. Data lineage refers to the history of data since it moves through different systems and processes within an organization. Data lineage understanding is important to ensure data compliance, data quality, and transparency, specifically in different AI applications. AI can automate the tracking of data lineage by analyzing data flows and making detailed lineage diagrams that visualize the transformation and movement of data across the organization. Specifically, this potential is crucial in complex environments where data is processed by different stakeholders and systems.
Modak delivers innovative data cataloging solutions that empower enterprises to fully utilize the potential of their data. Our skill sets lie in generating comprehensive data catalogs that not only manage and organize metadata but also improve governance, data discoverability, and compliance across different platforms. Implementing advanced artificial intelligence and machine learning tools, Modak automates lineage tracking, metadata generation, and data classification, making it effortless for businesses to maintain data integrity and quality. With our deep understanding of AI-driven analytics and cloud-native technologies, we help firms optimize their data management approaches, ensuring that metadata becomes a very robust enabler of business insights and operational efficacy.
Looking Ahead
As we look into the future, in AI-driven enterprises, the role of data cataloging and metadata will only grow. Evolving technologies like metadata generation, advanced search abilities, and automated data lineage tracking are set to transform the way firms use and manage their data assets. These innovations will make metadata management more scalable, effective, and integrated with AI systems, further improving the value of data in the organization.
But there are challenges along the way to effective metadata management and data cataloging. Enterprises must invest in the correct technologies, tools, and talent to make and maintain strong data catalogs. Also, they must establish a culture of data stewardship, where metadata is considered an important component of the organization’s data strategy. Finally, enterprises must stay updated on the innovations in AI and metadata management, evolving their practices continuously to lead the curve.
On data cataloging and metadata management, the transformative power is profound, paving the way for more innovative and effective data practices. Since firms continue to create and rely on huge quantities of data, the role of AI to manage this data becomes more necessary. Adopting AI in data management is not only regarding keeping up with technology-but also it is regarding the speed of innovation and efficacy in the digital era.