In the dynamic realm of life sciences, Artificial Intelligence (AI) stands as a game-changer, redefining the landscape of drug discovery with unprecedented possibilities. AI's transformative influence in deciphering biological complexities and accelerating scientific endeavours has ushered in a new era of innovation.
At the forefront of this evolution are two groundbreaking components: Generative AI (GenAI) and Large Language Models (LLMs). These pioneering technologies have swiftly gained recognition for their instrumental roles in revolutionizing life science research.
GenAI is a subset of artificial intelligence that focuses on creating, designing, or generating new content or information, often in creative or novel ways. It uses algorithms and models to produce new data, such as images, text, audio, or even video, mimicking human-like creativity and innovation.
On the other hand, LLM is a subset of Generative AI (GenAI) focused specifically on language-related tasks. While GenAI encompasses a broader scope of AI models that generate content across various formats like images, music, and text, LLMs are specifically tailored to understand, analyze, and generate human language. They are a crucial component of GenAI, specializing in language-based applications, such as natural language understanding, text generation, translation, summarization, and sentiment analysis.
GenAI's capabilities in analyzing large-scale patient data facilitate the development of personalized treatment approaches. It aids in tailoring medications based on individual genetic profiles, leading to more precise and effective therapies. LLMs excel in parsing and comprehending extensive scientific literature, patents, and biological data. Their ability to extract insights, identify patterns, and interpret complex data fosters a deeper understanding of diseases, biomarkers, and potential treatments.
Despite notable advancements, life sciences face critical hurdles in data integrity, ethics, and understanding AI solutions, necessitating careful consideration in this evolving landscape. The following challenges pose significant considerations demanding meticulous attention within the evolving landscape of scientific innovation and technological leaps.
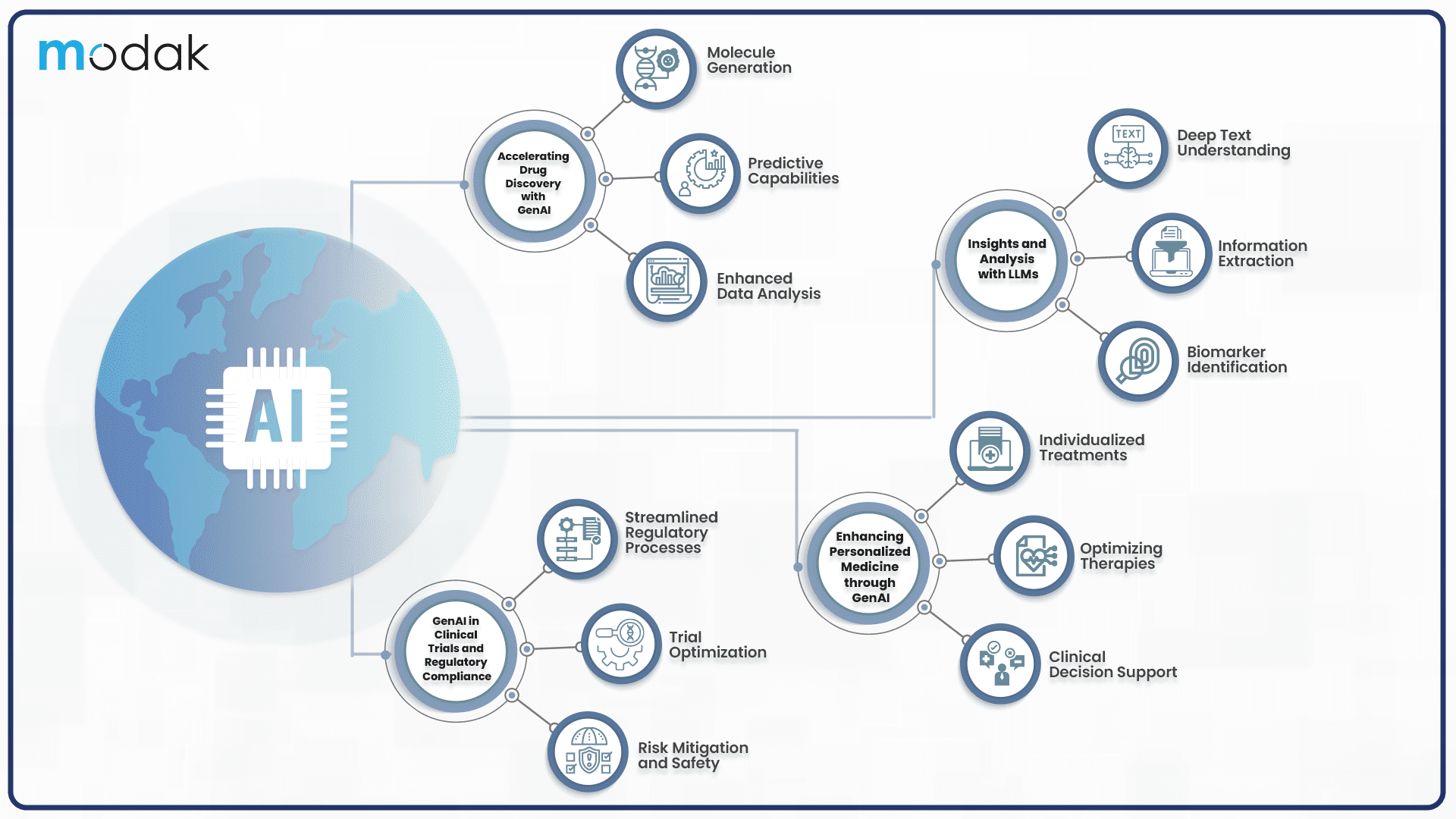
Challenges with GenAI and LLMs
Data Quality:
High-quality, diverse, and well-annotated data is the backbone of AI models. Ensuring data accuracy, relevance, and representativeness poses a significant challenge. GenAI and LLMs require vast, clean datasets for training to produce accurate and reliable outcomes. Insufficient or biased data could result in flawed predictions or reinforce existing biases, impacting the credibility and effectiveness of AI-driven insights and outcomes in life sciences.
Data Privacy:
Protecting sensitive information remains a paramount concern for businesses in an era defined by rapid digital transformation. Balancing the influx of innovative technologies with the imperative to secure personal, customer, and proprietary data poses an ongoing challenge for organizations. As the digital landscape continually evolves, ensuring the confidentiality and integrity of this information has become an ever-pressing priority. Along with data privacy issues, the ethical use of AI in life sciences demands meticulous attention to data privacy, consent, and fairness. Issues surrounding patient privacy, consent for data usage, and the potential biases ingrained within AI algorithms raise ethical dilemmas.Limitations of LLMs:
An inherent limitation plaguing LLMs lies in their contextual grasp of enterprise-related queries. These models, exemplified by GPT-4 and BERT, draw their extensive knowledge from publicly accessible internet texts, covering diverse subjects and fields. Yet, the training of enterprise GenAI models on internal data by accessing enterprise databases or exclusive data repositories poses a challenge. This limitation leads to fabricated responses or accurate but misplaced information that lacks relevance to the specific context.Interpretability:
The lack of interpretability in AI models, especially complex deep learning algorithms, poses challenges in understanding the rationale behind their decisions. In critical applications like healthcare, where transparency is crucial, the inability to explain why AI arrives at specific conclusions could hinder acceptance and trust. Ensuring the interpretability of AI outputs becomes vital, allowing stakeholders to comprehend and validate AI-driven insights and decisions, particularly in sensitive areas like diagnosis or treatment recommendations.Striking a balance between harnessing the potential of AI and ensuring ethical, transparent, and interpretable practices remains pivotal for the successful integration of GenAI and LLMs in life sciences.
As these pioneering AI technologies redefine the boundaries of research and innovation, organizations must navigate the intricate landscape of data quality, ethical implications, contextual limitations, and interpretability hurdles. Tackling the above-mentioned challenges proactively enables enterprises to harness the transformative capabilities of GenAI and LLMs while upholding ethical standards and enhancing the reliability of outcomes in healthcare and research.
Data Quality Improvement:
- Invest in Data Governance: Establish robust data governance frameworks ensuring data accuracy, completeness, and consistency across all stages.
- Data Cleaning and Enrichment: Employ data cleaning tools and methodologies to eliminate inconsistencies and biases while enriching datasets with relevant information.
- Collaborative Data Collection: Foster collaborations with academic institutions or industry partners to access diverse and high-quality datasets for training AI models.
Data Privacy and Ethical Considerations:
- Compliance with Regulations: Ensure compliance with data privacy regulations such as GDPR and establish stringent data security measures.
- Ethical AI Frameworks: Develop and adhere to ethical AI frameworks that prioritize fairness, transparency, and accountability in data usage and algorithmic decision-making.
- Transparency and Consent: Emphasize transparency in data collection and usage, securing explicit consent for the utilization of personal or sensitive information.
Contextualizing LLMs for Enterprise Queries:
- Customized Training Data: Train LLMs on enterprise-specific data, incorporating internal knowledge bases or proprietary information to enhance contextual understanding.
- Fine-Tuning Models: Employ techniques like transfer learning or fine-tuning models to adapt pre-trained LLMs to enterprise-specific contexts.
- Develop In-house Expertise: Build in-house expertise to curate and prepare data that aligns with enterprise requirements for training AI models.
Enhancing Interpretability:
- Human-in-the-Loop Approaches: Implement human-in-the-loop methodologies where human experts validate and interpret AI outputs, ensuring alignment with domain expertise and reinforcing trust.
By focusing on improving data quality, adhering to ethical guidelines, customizing AI models to enterprise contexts, and enhancing interpretability, enterprises can navigate the challenges and maximize the potential benefits of integrating GenAI and LLMs in life sciences responsibly.
With GenAI's ability to generate novel molecular structures and LLMs' prowess in mining vast repositories of scientific data, the stage is set for a profound metamorphosis in drug discovery methodologies.
Accelerating Drug Discovery with GenAI:
The role of GenAI in drug discovery is pivotal. It utilizes machine learning algorithms to generate new molecular structures, optimizing compounds, and predicting potential drug-target interactions. For instance, GenAI expedites molecule design by analyzing vast chemical databases and proposing novel compounds that show promise in therapeutic applications. Statistics and case studies reveal substantial time reductions and cost savings achieved through GenAI-led drug discovery efforts.
Insights and Analysis with Large Language Models (LLMs):
LLMs are instrumental in extracting insights from copious scientific literature, patents, and biological data. They aid in predicting drug side effects, identifying biomarkers, and interpreting complex biological data, allowing researchers to delve deeper into understanding diseases and potential treatments. Specific instances highlight how LLMs have transformed information extraction and analysis in life sciences, empowering researchers with unprecedented knowledge.
Enhancing Personalized Medicine through AI:
GenAI and LLMs contribute significantly to personalized medicine by analyzing patient data, including genetic profiles and health records. AI assists in predicting patient responses to specific medications or therapies, enabling tailored treatments based on individual characteristics. Examples showcase how AI-driven personalized medicine revolutionizes patient care and treatment outcomes.
AI in Clinical Trials and Regulatory Compliance:
The utilization of AI, particularly LLMs, in clinical trials and regulatory compliance streamlines processes, including drafting regulatory documents, ensuring compliance, and analyzing trial data. AI has the potential to reduce errors, expedite approvals, and enhance overall efficiency in the drug development pipeline.
In a nutshell, while challenges exist in implementing GenAI and LLMs in life sciences, concerted efforts and advancements are shaping a promising future. Addressing challenges in data quality, ethics, and interpretability while leveraging AI's potential will lead to transformative advancements, revolutionizing life science innovations for the betterment of healthcare industry and humanity.
About Modak
Modak is a solutions company dedicated to empowering enterprises in effectively managing and harnessing their data landscape. They offer a technology, cloud, and vendor-agnostic approach to customer datafication initiatives. Leveraging machine learning (ML) techniques, Modak revolutionizes the way both structured and unstructured data are processed, utilized, and shared.
Modak has led multiple customers in reducing their time to value by 5x through Modak’s unique combination of data accelerators, deep data engineering expertise, and delivery methodology to enable multi-year digital transformation. To learn more visit or follow us on LinkedIn and Twitter.